Introduction: current challenges for security operations centers
The number of cyberattacks on organizations around the world is growing every year. According to our data, successful attacks on organizations in 2023 increased by 23% compared to the previous year. Damage caused by attacks is also increasing. According to IBM, the average cost of data breaches worldwide reached a record high in 2023, showing a 15.3% increase compared to three years earlier. The total damage caused by cyberattacks, including complete or partial business shutdown and infrastructure recovery, grows by 15% annually and may reach about $10 trillion by 2025.
The rise in attacks on companies happens for various reasons. There's a trend toward total digitalization: many industries keep automating their processes and implementing new technologies. As a result, the number of devices on the external and internal perimeters is growing. According to a study by JupiterOne, the total number of cyber assets involved in business processes shot up by 133% in 2023. At the same time, malicious tools are becoming more automated due to artificial intelligence. This lowers the bar for attackers and gives them more opportunities, such as using open-source data reconnaissance, finding ways for malware evasion, or creating deepfakes and phishing emails. As a result, SOCs are struggling to keep up with the increasing workload. According to a Devo report, 78% of SOC staff work overtime.
There are various ways to gauge how well (or not so well) a SOC team is handling the increasing number of cyberattacks. For instance, based on the number of processed alerts from security tools. A study by Vectra AI shows that an average SOC team receives 4484 alerts every single day. According to Positive Technologies, an analyst needs about 10 minutes on average to analyze one alert. Therefore, no more than 100 alerts can be handled by one person in a 10–12 hour shift. Let's look at how many additional analysts are required and try to estimate how much financial resources companies need to employ them, taking into account the average salary according to the InfosecJobs portal.
Number of alerts | Minimum number of required SOC analysts | Payroll budget |
1,000 | 10 | $85K x 10 = $850K |
5,000 | 50 | $85K x 50 = $4.25M |
10,000 | 100 | $85K x 100 = $8.5M |
100,000 | 1,000 | $85K x 1,000 = $85M |
1,000,000 | 10,000 | $85K x 10,000 = $850M |
The second way to estimate the required number of SOC analysts is by the time spent working on one incident. Even though different incidents require varying amounts of time, the SOC Market Trends Report by the Enterprise Strategy Group shows that the average time spent on a single incident is three hours (with an average of six cybersecurity tools involved). According to Morning Consult, analysts spend an average of 32% of their time reviewing incidents that pose no real threat to the business.
Number of incidents per shift | Minimum number of required SOC analysts | Payroll budget |
4 | 1 | $120K |
12 | 3 | $360K |
24 | 6 | $720K |
48 | 12 | $1.44M |
96 | 24 | $2.88M |
Knowing your number of alerts or incidents, you can roughly estimate how many SOC analysts and what size payroll budget you would need (not considering backup analysts, weekend and night shifts, or sick leave, which may require doubling or tripling their number).
But even if you have the necessary number of SOC analysts, a large part of their time is spent inefficiently. According to Vectra AI, 83% of all security alerts are false positives, but processing them wastes precious time, preventing specialists from handling events that could signify a real cybersecurity incident. At the same time, the SANS 2023 Incident Response Survey shows that false positives have increased in recent years, from 75.9% in 2019 to 95.8% in 2023. According to Morning Consult, SOC analysts can't process 49% of cybersecurity events per day. This leaves 97% of analysts feeling uneasy and uncertain about their work outcomes, which often leads to burnout.
So how can SOCs cope with the growing challenges of cybersecurity monitoring? The most viable solution is to automate all detection, investigation, and incident response processes, aiming to reduce the amount of manual work.
Next, our study delves into why conventional SOCs always seem to be understaffed. Then we'll look at the levels of cybersecurity monitoring automation available today and reflect on what we can expect from a SOC in the future.
Why traditional SOCs are inefficient
In conventional SOCs, there are several factors that theoretically decrease the effectiveness of incident detection and response. First and foremost, they include:
- Insufficient visibility of the ever-changing IT infrastructure and potential attack surface
- Lack of context for analyzing cybersecurity events
- Dependence on timely delivery or development of detection content
- A large number of telemetry tools and sources that are not integrated with each other, which complicates the investigation process and increases decision-making time
- Difficulties in interacting with IT departments, delaying incident response
- The need for extensive logging of sources in the infrastructure (to increase the chance of detecting malicious activity), leading to a large volume of cybersecurity events, including false-positive alerts
As a result, SOCs take longer to detect and respond to cybersecurity incidents, while infrastructure monitoring suffers from blind spots. According to a 2021–2023 study by Positive Technologies Expert Security Center (PT ESC), an attacker is able to persist undetected in a compromised infrastructure for an average of 45 days. Meanwhile, the time it takes for attackers to conduct an attack is reducing dramatically. According to a recently published Palo Alto study for 2023, the time between an attacker's initial access to the infrastructure and the extraction of sensitive data dropped to a few hours and was less than one day in 45% of cases.
Caught in the spate of mass attacks, partly fueled by rapidly evolving AI tools, analysts risk missing targeted attacks that are becoming harder to detect. Over the past few years, for example, attackers have been actively using the living-off-the-land approach: after breaching the network perimeter, intruders use legitimate in-house tools of the compromised system to progress their attack and advance within the network. Such techniques are quite difficult to detect because attackers' activity masquerades as that of legitimate users. To address this challenge, modern SOC tools should at least have built-in behavioral analysis features based on AI algorithms. According to the Devo SOC Performance Report, 55% of respondents said that the lack of ML-enabled attack detection algorithms was the primary reason for SIEM inefficiencies in their SOCs.
Despite the variety of SOC tools designed to automate incident detection and response, some key processes in SOCs are still not automated. Analysts often have to do manual work such as connecting new telemetry sources, gathering incident context across multiple sensors, the number of which ranges from three to eight in 68% of cases, identifying chains of illegitimate activity in the infrastructure, and generating incident response scenarios. In fact, every stage of incident detection and response has such manual tasks that significantly increase the workload of SOC analysts. We compiled a list of such complex tasks and outlined their consequences at each stage (see Figure 1).
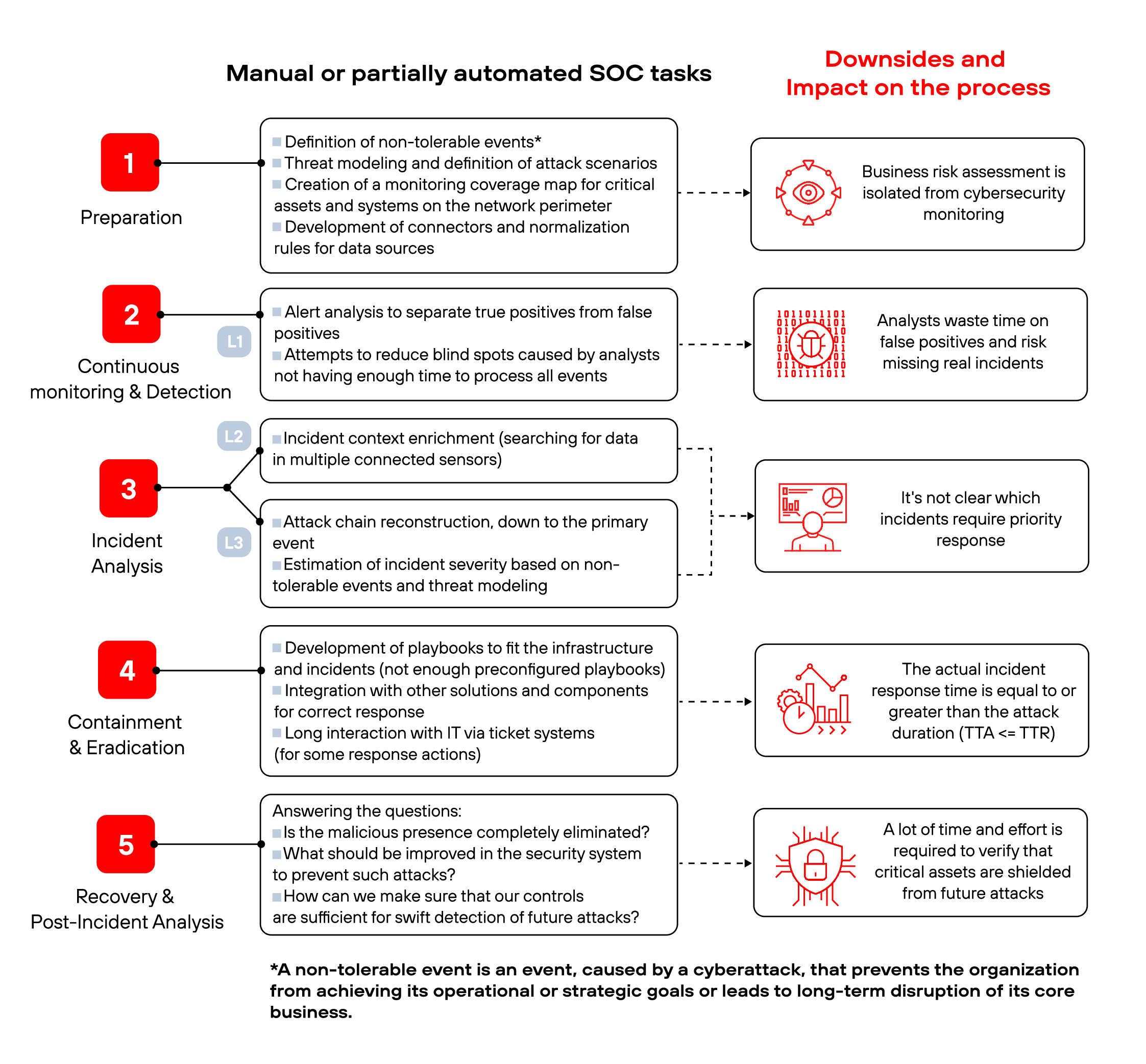
According to a SANS survey, more than 40% of companies realize the need to automate their incident response processes and infrastructure recovery after cyberattacks. The challenges facing incident response teams brought about the idea of a next-generation SOC that could address all the current complexities. Now let's take a look at our expectations and requirements from a next-generation SOC.
NG SOC: what we expect from a next-generation monitoring and response center
What is an autonomous SOC
The main feature of a next-generation SOC (NG SOC) should be its autonomy, that is, its ability to detect, investigate, and respond to incidents without human involvement. Therefore, an NG SOC can be also called an autonomous SOC. There is no official definition of an autonomous SOC as yet, and leading cybersecurity players each have their own way of describing it. Having analyzed more than a dozen different definitions, we can come up with a common description:
An autonomous SOC is a system (or a combination of tools) that provides continuous automated monitoring, detection, response to, and prevention of cybersecurity incidents, using machine learning algorithms and data analysis without human intervention.
This raises the question of how the automation level of SOC process can be measured. Is it possible to build a fully autonomous SOC in one go or is it a long, step-by-step endeavor? The closest analogy we can draw is autonomous vehicles and the SAE's levels of driving automation. We previously explored this question in another study where we identified parameters that can be used to describe the different stages of SOC automation. In total, we have identified 5 levels of automation, where level 1 means fully manual analysis of events from security logs, and level 5 means a fully autonomous cybersecurity monitoring and incident response process. See Appendix 1 for more details on SOC automation levels.
Like vehicles, SOCs have a long way to go when it comes to evolving from manual log analysis to full autopilot. Autonomy means a high level of automation, which is achieved by incrementally building and integrating the necessary security operations tools. Similar to vehicles, the difficulty in achieving Level 5 (full autopilot) is to enable the machine to navigate on its own in an unknown environment when there is no map of the terrain and no predefined situational patterns or action plans. To translate it into cybersecurity language: how will an autonomous SOC behave in case of a complex and previously unknown attack vector that employs new, hard-to-detect malware?
Features of an autonomous cybersecurity monitoring center
A classic incident response center consists of a number of components:
An autonomous SOC, while generally increasing the level of process automation, still continues using the well-known functional components at its core. On top of that, new modules or individual products are added to close automation gaps and gradually reduce the human workload. Let's examine in detail what needs to change in SOC tools.
Automated integration of telemetry sources
The first challenge during the SIEM implementation phase is the need to integrate multiple telemetry sources, which are not always supported by built-in connectors and require manual connector creation. However, some vendors are already developing capabilities to automate the writing of normalization rules. This is a complex task: first of all, you need to determine how much data to collect from a source is enough to be able to detect malicious activity, without being excessive. Second, some cases require conversion of source data into a format suitable for the SIEM solution. To solve these issues, you could create a SIEM module that automatically analyzes the formats used by various telemetry sources, marks up the source data, and transfers the resulting data to the SIEM system. Solutions to this complex problem are already emerging. For example, Israeli startup Avalor has created an AnySource Connector module that can collect data in any format from any source. Fast connection of new telemetry sources is achieved by the ability to work with any unstructured or unindexed data. For this purpose, artificial intelligence algorithms are implemented in the SIEM system.
Automated processing of false-positive alerts and typical events
Another important capability of an autonomous SOC is automated processing of a vast number of alerts, many of which are in the analyst's blind spot, and eliminating false positives. What mechanisms need to be implemented to meet this challenge?
Typically, analysis of event logs fed into a SIEM system is based on correlation rules. Correlation rules are based on the "if A, then B" logic, which allows events to be categorized as suspicious and generate a security alert. Attacker's activity in a compromised infrastructure is often similar to legitimate user activity (the living-off-the-land principle). As a result, some harmless user actions often trigger the correlation rules, causing the SIEM system to generate plenty of false-positive alerts. MaxPatrol SIEM, for example, uses an automatic whitelisting mechanism to streamline the processing of alerts triggered by actions of regular users: if an alert is categorized as false-positive, every subsequent instance of this alert will be automatically included into the list of exceptions and marked as a false positive.
To reduce the number of SIEM alerts, some analysis operations can be delegated to EDR/XDR solutions, which can analyze events on end devices and feed only true alerts to the SIEM system. To reduce the volume of transmitted telemetry logs, you can use tools that compress data and remove duplicates. These tools are integrated in the data transmission chain from cybersecurity sensors to data warehouses or a SIEM system. Also, we see the emergence of technologies for intelligent compression of event logs based on time intervals. This approach is implemented in the LogSlash solution, for example.
Correlation rules aren't going anywhere just yet; they remain one of the primary mechanisms for analyzing event logs and a way to supply SIEM solutions with analytical expertise. However, there is an emerging opportunity to use AI to assess the potential of correlation rules. For example, LogRhythm now offers a risk-based priority (RBP) calculator that estimates the probability that a rule will generate a false positive. At the same time, AI-based solutions are emerging that learn from incident history and can quite accurately assess SIEM alerts as to whether they are true or false positives. For example, the automated responder knowledge (ARK) module in Sumo Logic analyzes alerts to identify true threats. Another example is the ML-enabled behavioral analysis module in MaxPatrol SIEM, called Behavioral Anomaly Detection (BAD), which is able to validate alerts triggered by correlation rules. BAD acts as a second opinion system, improving attack detection by providing an alternative method of evaluating security events.
There is, however, another approach to eliminating false-positive alerts, which is based on data enrichment algorithms that examine causal relationships between multiple cybersecurity events. For example, MaxPatrol O2 organizes all incoming events by resource (nodes, sessions, processes, services, files, tasks, or accounts) and analyzes them based on knowledge of attack patterns. In doing so, MaxPatrol O2 can determine which node or process performs malicious actions and which is the target of malicious activity. This approach makes it possible to distinguish logically connected chains of high-severity malicious actions from other SIEM alerts, thereby filtering out and eliminating false-positive alerts at the stage when connected events are glued together.
Implementation of behavioral analysis algorithms
Correlation rules and malware signatures help detect known attack patterns and are quite effective in accomplishing such tasks. But when it comes to detecting unknown behavior and abnormal activity, behavioral analysis algorithms come into play. UEBA functionality is offered in almost every solution that claims to be an autonomous SOC or Autonomous Security Operations Platform. The value of behavioral analysis systems is that they detect threats based on abnormalities in user behavior, such as atypical processes being launched or unusual files being opened. This allows you to more accurately determine if some potentially malicious activity is behind a user's actions.
UEBA systems employ AI extensively, with each vendor implementing behavioral analysis in their own way. Here are some examples of algorithms used in UEBA systems:
- Clustering. In particular, the k-means method can categorize all users into behavioral groups, and the k-nearest neighbors method compares the behavior of a new user with that of other users and determines whether this user is similar to certain user groups.
- Support vector machines (SVM). An algorithm finds classification boundaries that separate harmless users from potentially malicious ones and determines whether a new user fits into these boundaries. SVMs work efficiently in high-dimensional spaces and can be used to classify security events as true or false.
- Converged neural networks are used to detect anomalies in network data. They allow you to find suspicious activity on your network, such as hacking attempts or attempts to spread malware.
- Collaborative filtering algorithms were originally designed for recommender systems. For example, the Behavioral Anomaly Detection (BAD) module in MaxPatrol SIEM uses these algorithms.
In addition to information about user and entity behavior, machine learning algorithms can leverage knowledge of adversary techniques used at different attack stages (such as execution, command and control, and lateral movement) to enrich behavioral analytics with expert knowledge. For example, the BAD module in MaxPatrol SIEM also integrates such knowledge of adversary tactics and techniques.
Automated context enrichment
The next step an analyst typically takes is gathering the context data on a particular alert triggered by a correlation rule or generated by the behavioral analysis module. This is called data enrichment and involves looking for events flagged by other connected sensors, such as NDR systems or threat intelligence (TI) platforms. The data enrichment step can take quite a long time.
How can the data enrichment process be automated? Again, different vendors choose different paths. When analyzing alerts triggered by correlation rules, SOC analysts spend a lot of time on reconstructing the sequence of interconnected process launches. This is necessary to decide whether a SIEM alert is true or false, because data about the parent process is often not enough to assess the situation. MaxPatrol SIEM, for example, uses a mechanism that automatically enriches any correlated process-containing event with a complete process chain and records this information in a separate field.
To analyze signs of malicious activity, multiple parameters may be required: account, host, running process, source IP address, destination IP address, and more. We can assume that AI algorithms will be able to create automated search filters for collected data and external sources, and use these filters to analyze data and produce enriched context data for cybersecurity events. For example, Elastic upgraded their search capabilities by incorporating vector transformation for unstructured data into their Elasticsearch Relevance Engine (ESRE) module. ESRE enables you to build highly relevant AI-powered search applications, giving developers a complete set of sophisticated search algorithms with the ability to integrate with large language models (LLMs). Search results can be collected from different sources and ranked for relevance using the Reciprocal Rank Fusion (RRF) method. Another example is Query, a federated search solution that searches all connected cybersecurity sources to find the answer to a given question.
Building attack chains: from detection to retrospective analysis
With AI, we can significantly reduce the number of alerts in SIEM systems, decrease the number of false positives, add context to information security events, and receive reliable incident alerts. The challenge then is to unfold the full attack chain to understand how the incident developed, where it started, how to stop it, and how to prevent the attacker's next steps. Normally, analysts handle this process manually, which requires a high level of expertise. Now, this task can be delegated to an autonomous SOC.
Algorithms can analyze connections between hosts, processes, accounts, and open sessions, identifying unusual interactions between objects that should not occur during normal system behavior. It is crucial not only to identify objects and entities with unusual connections, but also to determine the lateral movement vector, identifying the network resource acting as an attacker, the resources compromised by the attacker, and which hosts are being scanned and targeted. To identify attack chains, we use data about assets within the protected infrastructure, knowledge of attackers' tactics, techniques, and procedures, and indicators of compromise integrated into algorithms. This functionality is already implemented in solutions such as MaxPatrol O2, Exabeam Fusion, and Hunters Security, which can perform automatic analysis and generate an attack history. Information about attack chains and the resources involved is presented in a graphical format that may include several subgraphs illustrating lateral movement vectors.
Automated building of attack chains implies that algorithms of an autonomous SOC understand network topology and have up-to-date information about protected resources. This information must be automatically delivered to the SOC.
Graphical representation of the infrastructure and dynamic asset mapping
For effective automated attack chain building, up-to-date information about network topology and assets is essential. Asset management system functions are integrated into an autonomous SOC to continuously receive information about network resources from sensors, analyze this data, find connections, and build an asset map that includes devices, users, software, applications, data, configurations, and more. The asset map should dynamically adjust to changes in the network, such as the introduction of new hosts, changes in system and network configurations, or the decommissioning of assets. Additionally, the latest vulnerability data from vulnerability management systems should be transferred to asset management systems, as vulnerabilities can significantly influence attack scenarios and must be tracked in real time. For example, PT MaxPatrol SIEM and MaxPatrol VM integrate information about infrastructure assets (asset management), vulnerabilities (vulnerability management), and compliance control (host compliance control). Together, they aggregate data from all sources into a single repository in an adapted format with the ability to generate PDQL queries.
Graph data analysis algorithms are used to create an asset map—a graphical representation of all collected information. It is vital that all SOC components function smoothly, with quick delivery of telemetry from sensors to asset management systems, efficient data analysis, identification of connections, and building of connection graphs. Then, we can apply attack scenarios to our dynamic asset map. In future, there is the potential for automatic blocking of forbidden or dangerous paths on the asset map. If we emphasize the most critical resources on the graph, we can assess how close an attack scenario is to triggering a non-tolerable event.
Simulating threats and calculating the reachability of critical hosts
Traditionally, threat assessments are presented as separate documents in which experts predict which threat scenarios can be realized in the infrastructure, considering its composition, architecture, and configuration. However, this approach is inefficient, as the infrastructure is constantly changing, meaning that new connections between assets and new attack paths appear regularly and need to be monitored, analyzed, and integrated into the information security system. Moreover, simply theorizing about attacks makes it difficult to build attack scenarios that fully cover attack paths as they would occur in real infrastructure, posing the risk of missing potential scenarios. Therefore, threat assessment also needs to be automated.
With a dynamic asset map, real-time attack chains, and information about the most critical resources in the network, an autonomous SOC can calculate the reachability of these resources from any point in the topology. When signs of malicious activity are detected, an autonomous SOC can estimate how many more assets attackers need to compromise, or in other words, how many steps are left to reach critical resources. This transforms attack chains into vectors, giving us an insight into how close the attackers are to their target.
With this approach, we can assess the severity of attack chains as a whole, not just isolated incidents. Assessment criteria may include how close an attacker has come to a target and the number of potential attack paths that pass through compromised hosts: the more paths an attacker has for pursuing an attack, the more severe the chain is. Such functionality is implemented in the Threat Modeling Engine (TME) module as part of the MaxPatrol O2 metaproduct. Some vendors of cybersecurity solutions also use other criteria when assessing the severity of an attack chain. For example, the Orca Security cloud security platform features the Business Impact Score (BIS) mechanism, which assesses several factors: the importance of a target to business (Business Impact), the severity of exploited vulnerabilities or security flaws (Severity), the accessibility of the entry point into the infrastructure (Accessibility), the risk of lateral movement from this entry point and the number of steps needed to reach the end goal (Lateral Movement Risk), and the level of access rights and privileges granted (Access Level). This assessment enables an autonomous SOC to prioritize attack chains by severity and suggest appropriate incident response scenarios.
Automated attack management: generating and running response scenarios
Thanks to artificial intelligence and machine learning technologies, an autonomous SOC can automatically generate incident response scenarios. These scenarios are based on the analysis of historical data and current events, allowing the system to learn from past experiences and adapt to evolving threats. Upon detecting an incident, the system automatically creates response scenarios that include a sequence of steps to neutralize the threat, based on the specifics of the detected incident and the assets at risk.
An autonomous SOC can automatically launch tasks and procedures to address the incident, such as isolating infected devices, deleting files, terminating processes and their child processes, and blocking accounts. By handling attack chains rather than isolated alerts, the SOC creates incident response scenarios designed to completely remove attackers from the infrastructure. This approach ensures a rapid response to incidents, reduces the time needed to mitigate threats, and minimizes potential damage to the infrastructure.
For example, MaxPatrol O2 can automatically generate response scenarios that can be run through MaxPatrol EDR agents. This innovative approach does not rely on the type of incident but rather on detailed information about the attacking, attacked, and compromised resources. Consequently, MaxPatrol O2 can generate a response scenario for any type of attack, whether it involves blocking a compromised domain account via the domain controller, blocking attackers through the firewall, or removing a malicious file from a host.
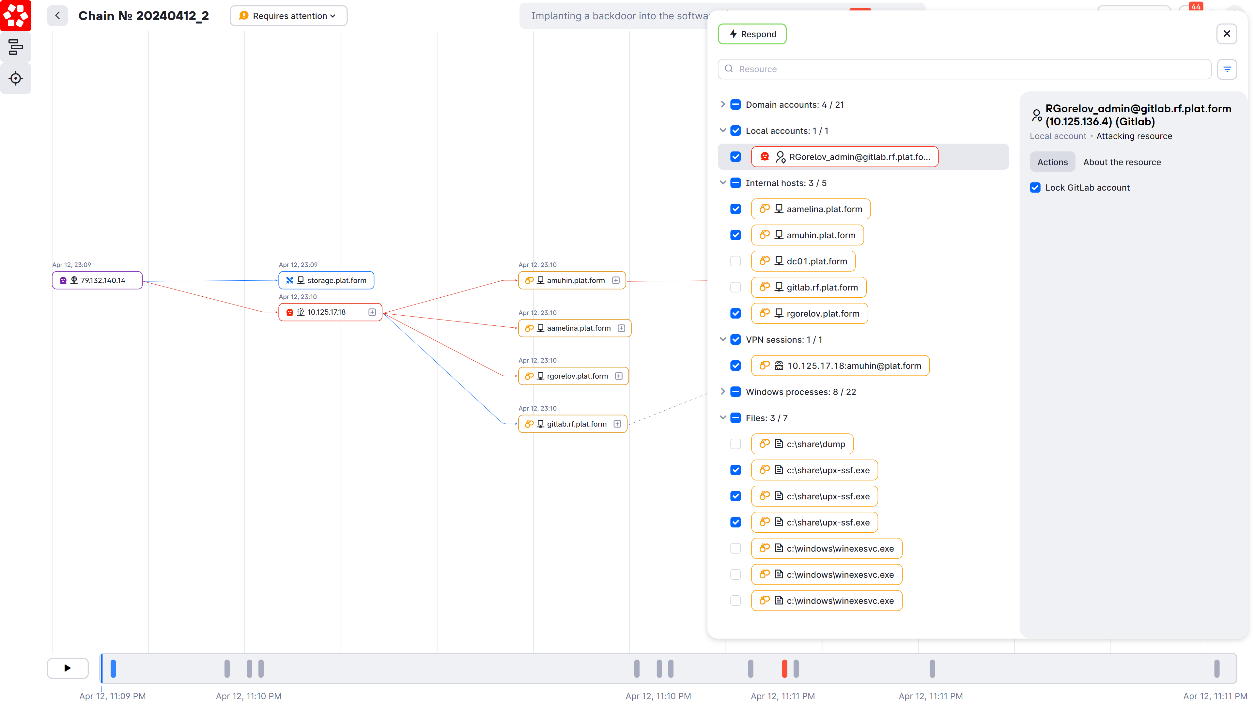
At the fourth level of SOC operations, human intervention may be required. Cybersecurity specialists review the generated playbook and make adjustments if needed. Nonetheless, an autonomous SOC significantly eases the workload of cybersecurity specialists, enabling them to concentrate on more complex tasks and strategic security management.
Built-in expertise
To accurately identify signs of malicious activity, build attack chains, assess their severity, and suggest response scenarios, an autonomous system requires built-in expertise. There are several methods to integrate this expertise into a SOC.
The most common method currently is through vendor expertise enhancement and the rapid delivery of detection content. Manufacturers of SOC tools must possess robust expertise, typically demonstrated by having their own divisions or subsidiaries dedicated to penetration testing and incident investigation. Experience gained from incident investigations enhances this expertise.
Another method involves SOC analysts writing new detection rules in a specialized console. In some next-generation SIEM systems, such as RunReveal and Cortex XSIAM, detection rules are written using Jupyter Notebook (or in Python for systems like Panther or Matano), adopting the detection-as-code approach. This involves the full-fledged development of detection rules, including testing and functionality checks.
An additional option is leveraging ready-made third-party expertise. Modules and connectors for various security tools, as well as new detection content—including content developed by other SIEM users in systems like MaxPatrol SIEM, Splunk, QRadar, and LimaCharlie—can be purchased on marketplaces. If SOC analysts are sufficiently qualified, freely distributed detection content in SIGMA format can also be used. The public SIGMA rule repository contains several thousand rules available for use.
Future autonomous SOCs may automatically create detection rules, using AI's capacity for self-learning and enriching existing expertise with new experiences. Eventually, intelligent systems capable of analyzing traffic and logs will emerge, identifying previously unknown attack patterns and incorporating new tactics, techniques, and procedures into the ATT&CK and ATLAS matrices.
Decision-making in an autonomous SOC
In an autonomous SOC, new tools are needed to tackle business-related tasks. These tools might evolve into a new class of cybersecurity monitoring tools, such as autonomous security operations platforms, extended security intelligence and automation management systems, as well as automated threat actor detection and response tools. Currently, no unified standardized class of solutions exists. Ultimately, no matter what you call it, it is essential to have a tool that can automatically detect incidents, build and investigate attack chains, identify critical attacks, and take response measures.
Decision-making can vary depending on the level of expertise and automation built into each product. Decisions may be made by a machine or an analyst. Regardless, analysts will handle significantly fewer incidents per day compared to thousands of isolated events managed by a traditional SOC.
Large Language Models (LLMs) and Generative AI (GenAI) will play a significant role in future SOCs. With access to vast knowledge bases and the ability to understand requests and respond in human language, GenAI can function as a copilot or even in autopilot mode. LLMs and GenAI can analyze large volumes of data from various telemetry sources and identify configuration errors (like Exabeam Threat Explainer), analyze and classify different types of threats and malware, predict potential cyberattack vectors (like LogRhythm AI Engine), generate threat response strategies (like Fortinet Advisor for FortiSIEM), and forecast possible outcomes and consequences of decisions made.
Evaluating the efficiency of a SOC
In a SOC, a set of metrics is used to reflect the time it takes to complete a full cycle, from detecting an incident to mitigating its consequences. Let's visualize all the known metrics on a timeline (Figure 4):
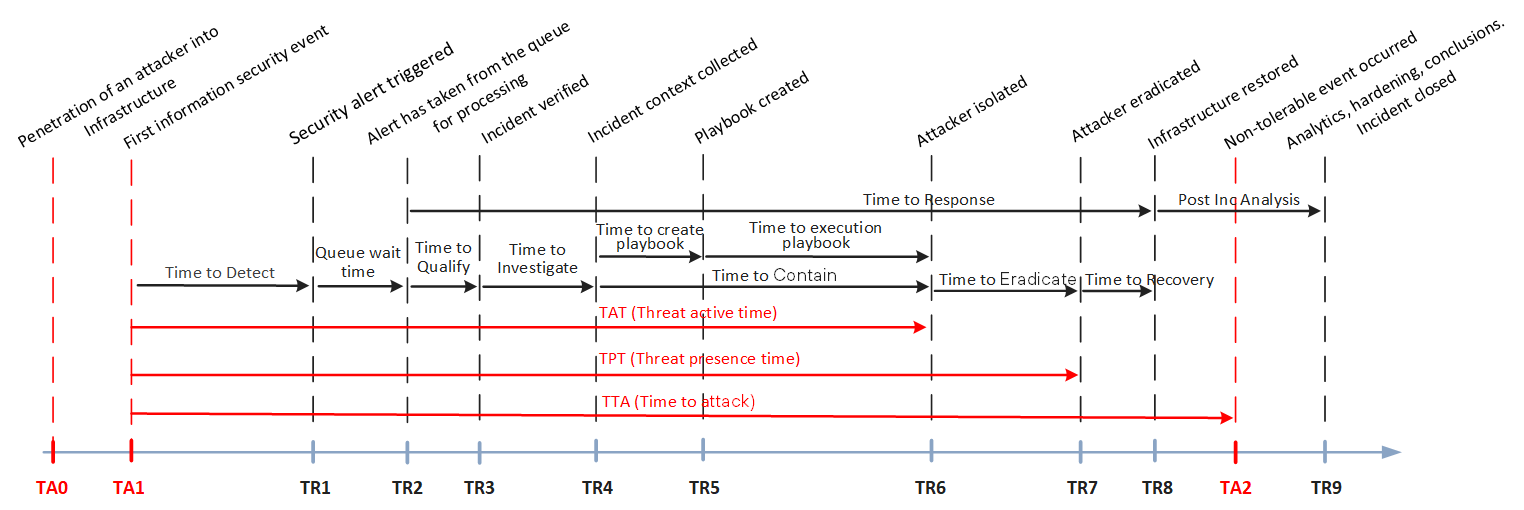
In a traditional SOC, when an alert is triggered, an analyst begins the analysis using key metrics. These include Queue Wait Time—the time until an alert is addressed from the queue—and Time to Qualify—the time needed to verify whether an alert signals a real incident. If the alert is confirmed as genuine, the investigation begins, measured by Time to Investigation. In contrast, an autonomous SOC works with attack chains instead of isolated security events, allowing for independent data enrichment and retrospective analysis of information security events. This reduces the queue of information security events awaiting human attention, significantly decreasing time-related metrics like Queue Wait Time, Time to Qualify, and Time to Investigation. As a result, there will be almost no delay between receiving an alert and obtaining complete information about the incident.
Similarly, the metrics Time To Contain (TTC) and Time to Eradicate (TTE) are approached differently in an autonomous SOC. In a traditional SOC, after verifying an incident, an analyst blocks attackers on the host where they were detected. Then, the analyst investigates which other devices or accounts within the infrastructure have been compromised and can be used to relaunch the attack. A response scenario is then prepared and executed, either manually or partially automatically (using XDR solutions) to eliminate attackers from the infrastructure. Now, let's consider how this approach changes in an autonomous SOC. The next-gen SOC automatically builds an attack chain from the point of incident detection to the point of attacker penetration in the network, and then creates a response scenario for the entire attack chain. Depending on the level of automation, the SOC either initiates a response scenario or waits for analyst verification. This means that the TTC and TTE metrics will tend to converge on the timeline (see Figure 6 above).
Eventually, traditional metrics will become less relevant for an autonomous SOC, and new efficiency metrics will be required. These will include tracking the progress of an attack (Time To Attack) and the progress of threat response (Time To Response) in real time. Instead of focusing on time, a SOC will consider the number of steps needed to trigger a non-tolerable event (more about this in another study). When comparing metrics, a SOC will ensure that the TTA >= TTR equation is not violated. The efficiency of an autonomous SOC will ultimately be measured by its ability to prevent attackers from causing a non-tolerable event, which can be assessed through regular cyber exercises.
Human in an autonomous SOC: are they needed or not?
When discussing automated incident detection and response, the question arises: what will be the role of humans once autonomous SOCs are implemented?
Even at the highest level of automation, human involvement remains necessary for tasks such as monitoring the system, making decisions in complex situations, running verification tests, identifying current threats, designing dashboards, and implementing and maintaining sensors. This mimics the use of autopilot in cars, where a human still oversees the algorithms' reactions to obstacles, road changes, weather conditions, and other variables. Similarly, a next-generation SOC must navigate new situations when previously unknown attack patterns and malicious tools emerge. In the early stages of autonomous SOC development, analysts are involved in a broad range of activities. Even at the fourth level of automation, human involvement is required to validate critical attack chains, verify and execute incident response scenarios. In addition, cybersecurity teams continue to engage in infrastructure hardening tasks, such as network device segmentation, managing user access rights and privileges, and addressing weaknesses in IT system configurations. The resources freed up by automation can then be redirected to these tasks.
The goal of an autonomous SOC is to prevent attackers from realizing critical risks, even in companies with a shortage of cybersecurity specialists. Autonomy does not imply that humans are unnecessary; rather, it means fewer are needed. A next-generation SOC addresses the acute shortage of SOC analysts, not by laying off staff but by reducing the number of man hours required for monitoring and handling incidents each day. Traditionally, the number of man-hours was fixed, calculated based on SOC operation conditions such as the number of protected assets, connected telemetry sources, and daily triggers in a SIEM system. In autonomous SOCs, cyberthreat management will require significantly fewer people. With the introduction of MaxPatrol 02, SOC efficiency increases by a factor of at least 30.
Conclusion
Autonomous SOCs represent the next step in the evolution of cybersecurity monitoring. The next-generation SOC is expected to solve many of the routine challenges faced by traditional SOCs, reduce Time to Response (TTR), improve analytics and decision-making, decrease analyst workloads, and remedy staffing shortages.
Let's review the key features of a next-generation SOC.
Automation at the highest level
To increase incident response efficiency, SOCs need tools that automate nearly all analyst actions. The system must understand the current network topology and asset map, be capable of building graphs of possible attacks, and request and analyze additional data from connected sensors. The automation of each subsequent task is closely related to the automation of the previous one, producing a synergetic effect that significantly accelerates incident detection and investigation.
Adaptation to game changes
Significant advancements in AI over the past few years have forever changed information security incident monitoring and response. Hackers increasingly use AI to discover entry points into infrastructure, as well as to craft phishing emails, exploits, and malware. The number of information security events is rising, as is the cost of not responding to them in a timely manner. We must embrace a new approach in which defenders also incorporate AI tools into daily information security monitoring.
Analytics and decision-making
The NG SOC will feature extensive built-in analytics and expertise required for decision-making. These analytics will rely not only on detection content received from external sources but also—and particularly—on the self-learning capabilities of AI algorithms. Thanks to machine learning and AI, next-generation SOCs will eventually be able to learn from previous attacks and adapt to new ones, which is particularly valuable in combating today's cyberthreats.
Autonomy
The NG SOC aims to achieve complete autonomy in information security monitoring and response. Previously, the development of incident response tools aimed at creating automation tools to assist SOC analysts (automation-assisted security operations). The current goal is to develop an autonomous system. Decisions made by this system will be checked and adjusted by an analyst if necessary (analyst-assisted security operations), enabling SOCs to analyze information security event data without human intervention, identify incidents, prioritize them, and propose relevant response actions.
About this study
The data and findings presented in this study are based on Positive Technologies own expertise, as well as analysis of publicly available resources, including government and international publications, research papers, and industry reports. Definitions of terms used in this report are available in the glossary on the Positive Technologies site.
Appendix
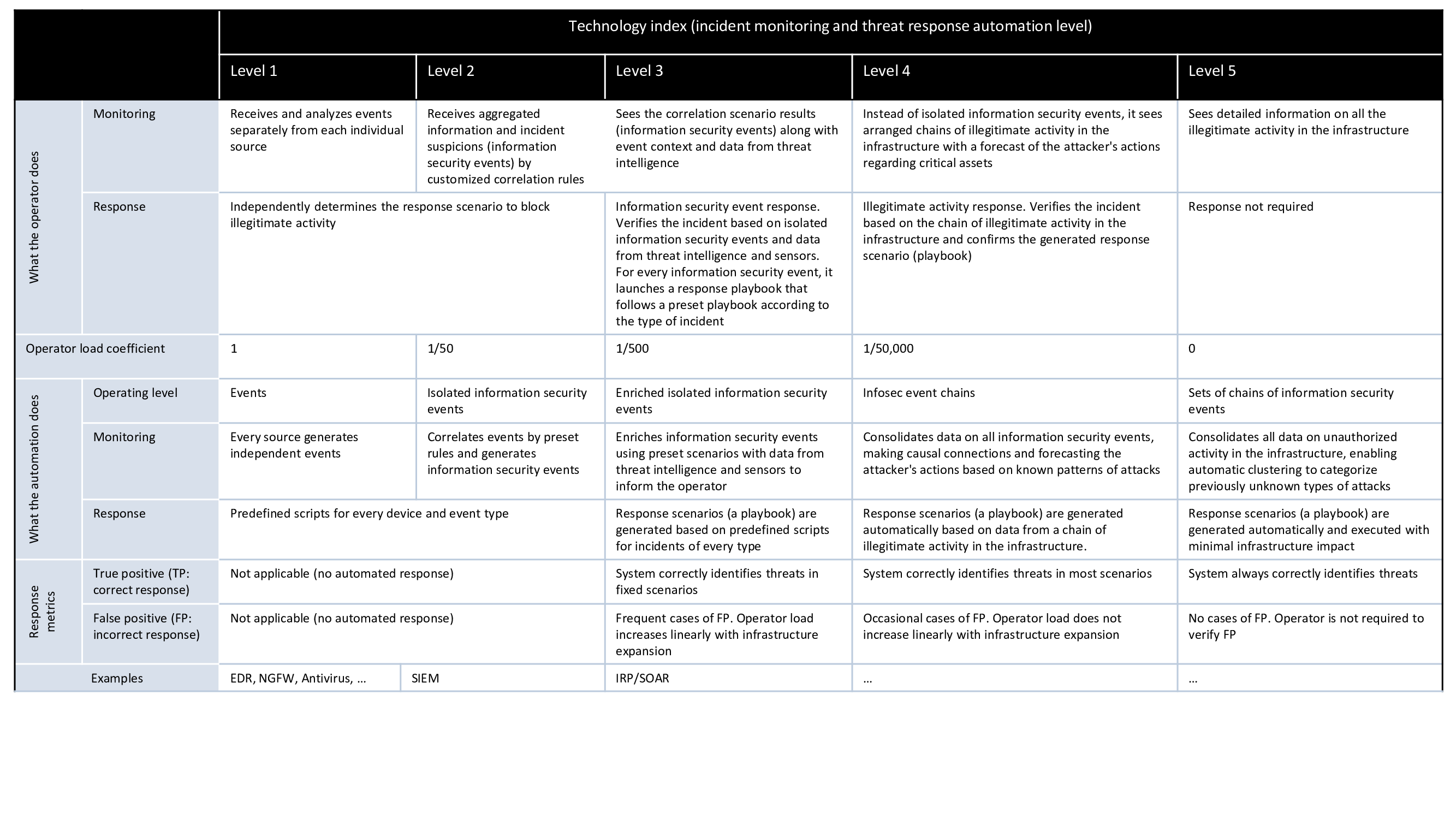
Table 3. SOC automation index
Table 3. SOC automation index
Get in touch
will contact you shortly